Apr 2024 Update:
Our paper, "A Learning Approach for Increasing AI Literacy of XAI in Informal Settings: Current State and Where to Go from Here" has been accepted at the AIED 2024!
The conference is the 25th Annual International Conference on Artificial Intelligence in Education. It will take place July 8-12, 2024 in Recife, Brazil and Virtually. It will be featured as a short paper at the conference.
Human-Centered Artificial Intelligence research about the use of cognitive learning objectives behind explainable AI (XAI) design conducted under Professor Iris Howley at Williams College.
This research is centered on understanding how XAI designers can design explainables as effective learning tools for intended users.
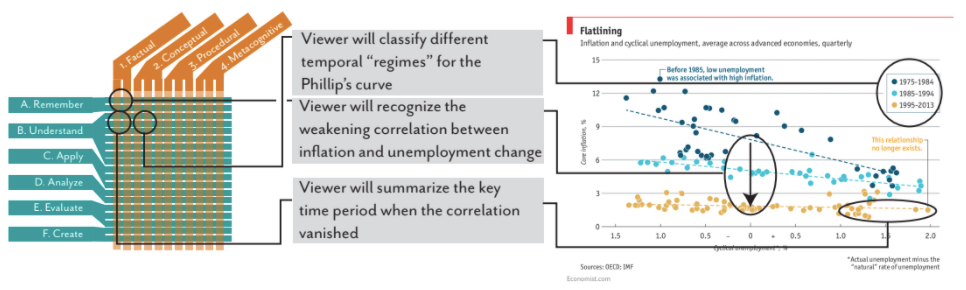
01 \\ What is an Explainable?
An explainable is an interactive tutorial that teaches users a specific concept. Explainables can come in varied forms, such as a game, data visualization, etc.
Knowing this, and heavily inspired by the article, “Communicative Visualizations as a Learning Problem” by Elsie Lee and her advisor, Eytan Adar, we wanted to investigate whether designers of explainables consider cognitive learning objectives when designing their explainables for users.
02 \\ Prior Literature
Bloom's Taxonomy of Learning
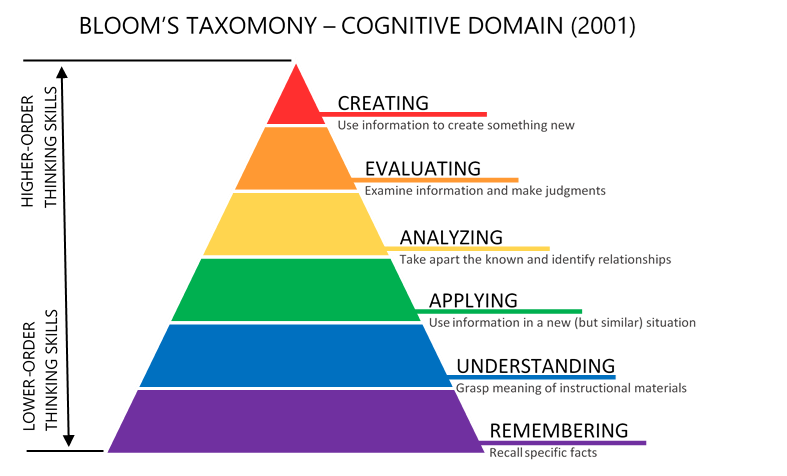
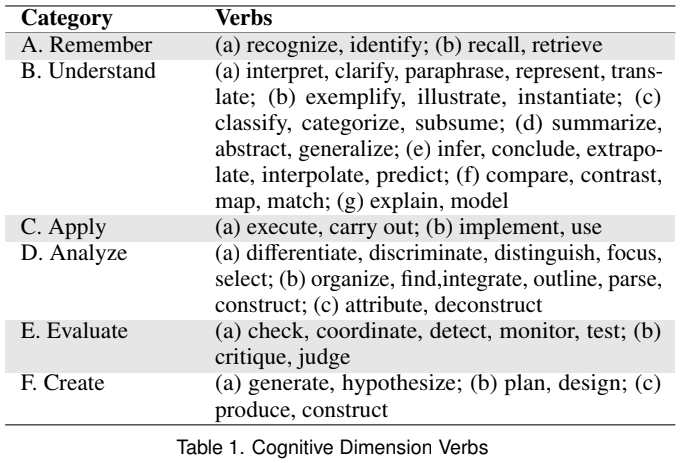
Backward Design
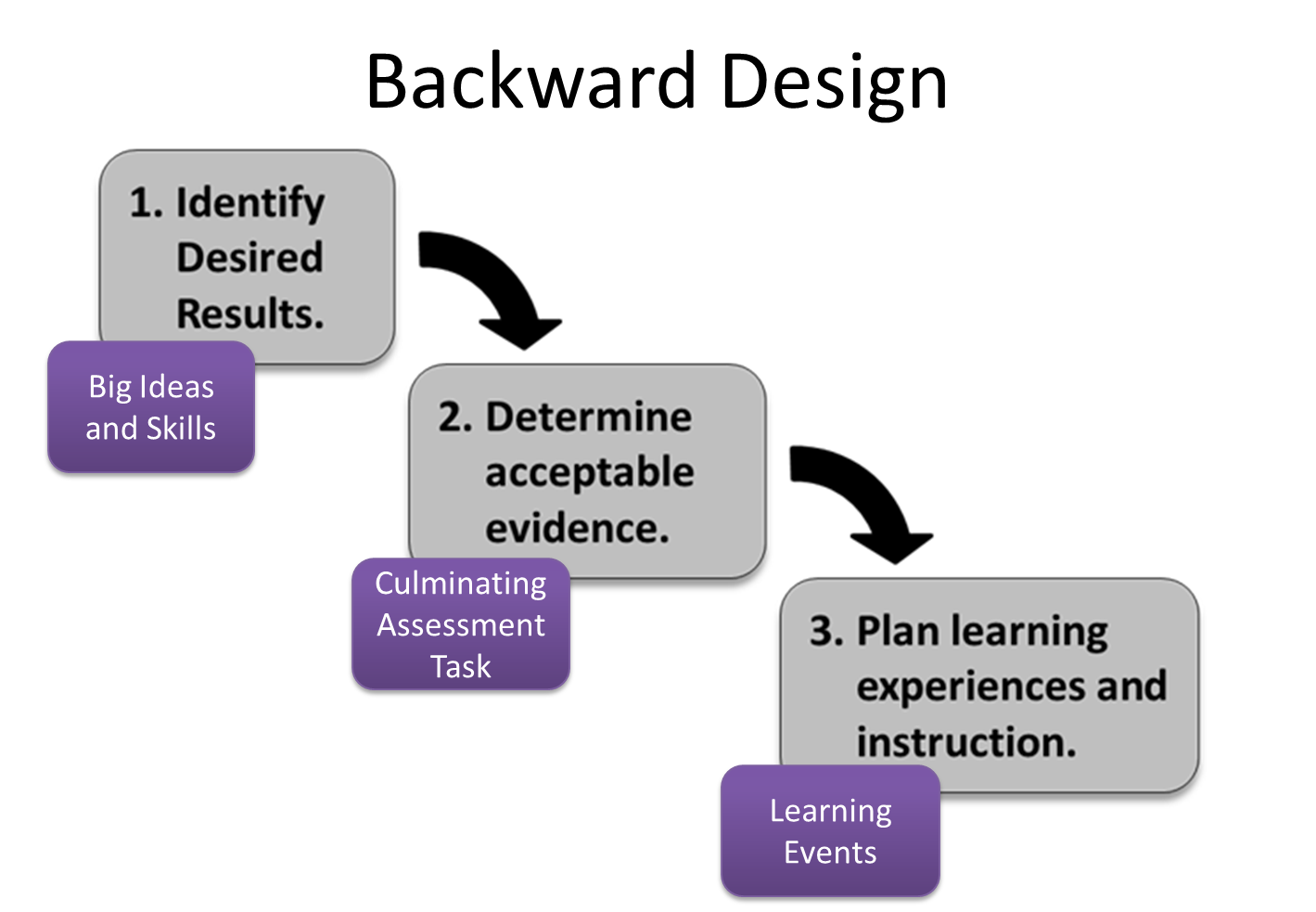
Knowing how there are three learning domains in teaching, as according to Bloom's Taxonomy of educational learning objectives, we decided to focus on the cognitive learning domain. This is because explainables invoke users' cognitive learning capacities by allowing them to gain mental skills and knowledge.
Therefore, we wondered whether designers consider cognitive learning objectives for their explainables prior to implementation. Considering cognitive learning objectives to map out desired results and then building the explainable project aligns with the design process called "Backward Design."
Backward Design is a process where designers identify the desired results of a product prior to designing the actual product. It is a popular process used by teachers in designing learning curriculum, so we want to see if this process is as effective for designing explainables, and if it is, should it be one employed by all creators moving forward.
03 \\ Research Design
We decided on writing personalized surveys that are personalized with specific cognitive learning objectives in a drop down menu for each designer's explainable. In addition to the surveys, creators will have the option of participating in a follow up interview where they can talk about their explainable and their design processes in greater depth.
We will compensate creators for their time after they complete the surveys, and if they were to participate in the optional follow up interviews. We estimate the surveys will take approximately 10 minutes and the interviews to take approximately 1 hour.
04 \\ Survey Design
We collected the contact information of creators who have ever participated in the Visualization for Explainability AI workshop, and those who created an explorable (projects synonymous to explainables) featured on explorabl.es/all/. I also took notes on these explainables to later guide how I write the personalized surveys.
When designing the surveys, I consulted the cognitive learning objectives taxonomy of learning verbs to create the personalized objectives within the surveys for each explainable. The surveys would be designed via Qualtrics and data will be analyzed with Tableau.
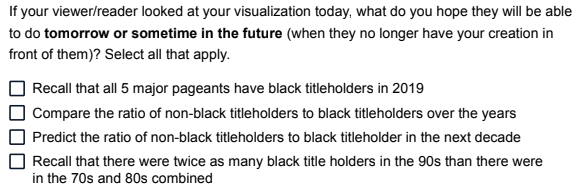
Sample survey with personalized cognitive learning objectives, created by Elsie Lee.
05 \\ Initial Testing and Feedback
I surveyed and interviewed two students in computer science who have designed and created their own explainables for preliminary testing and feedback.
From them talking through their responses when answering the surveys and talking through their design process and asking questions whenever clarification was needed, I was able to edit and improve my survey design and interview questions before conducting the actual research.
06 \\ Final Survey and Interview Questions
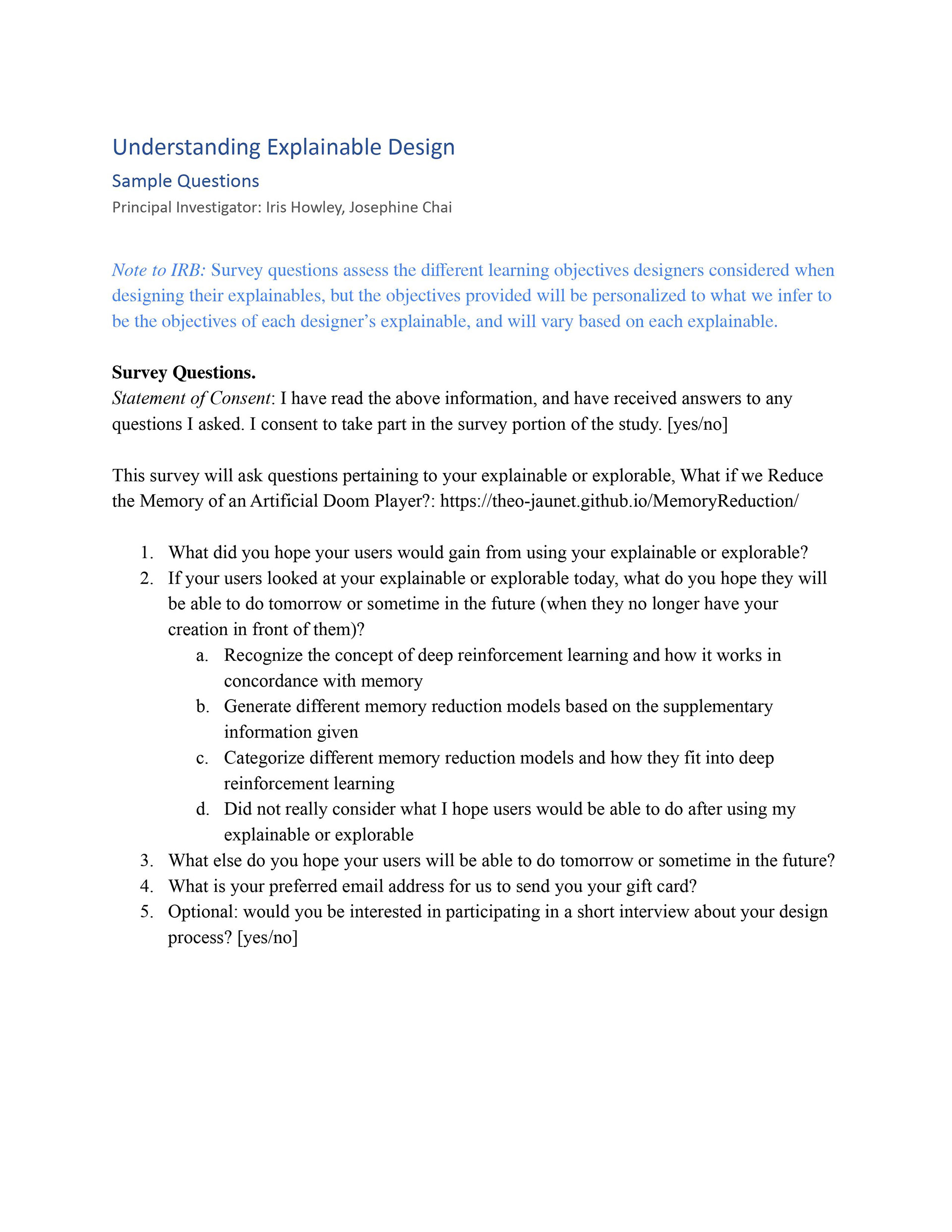
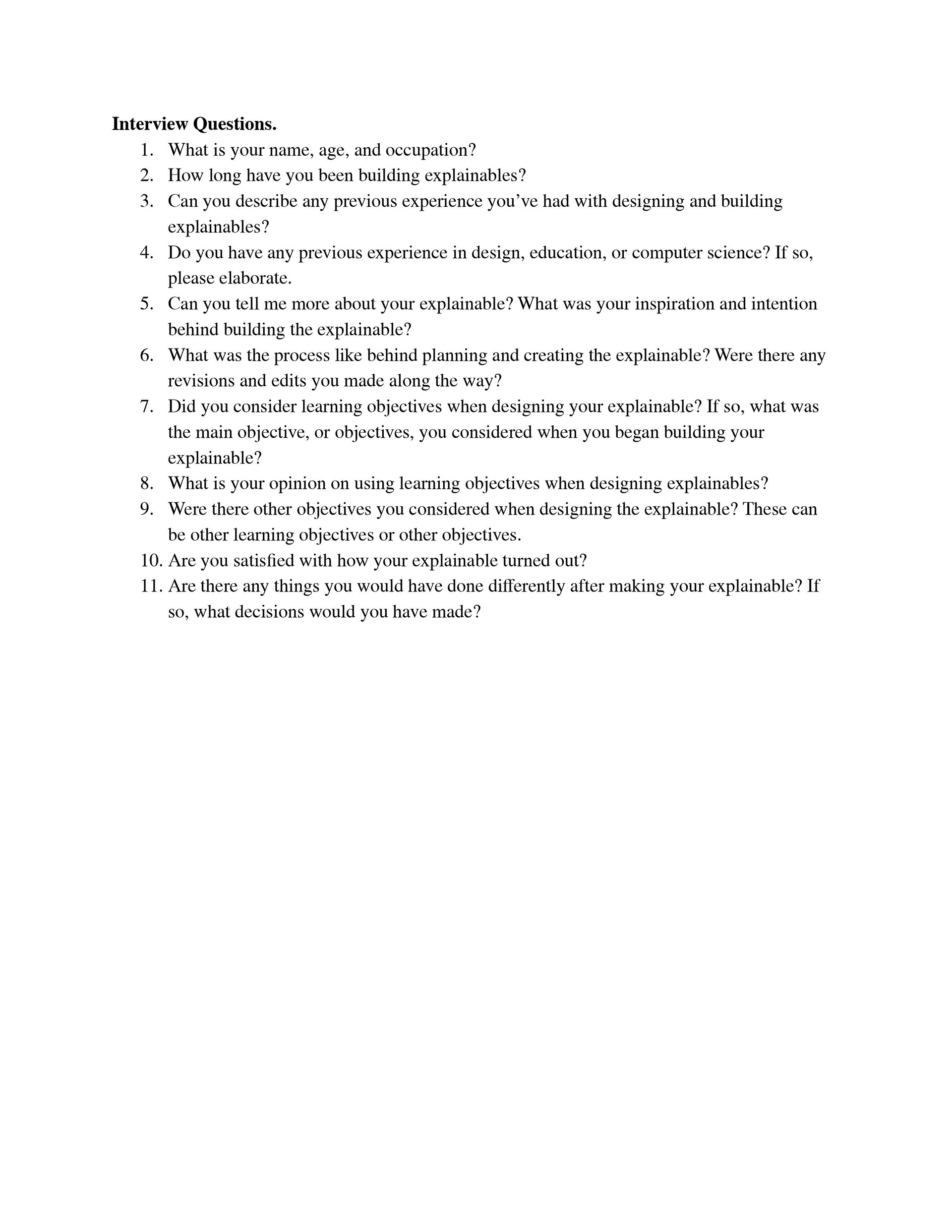